All published articles of this journal are available on ScienceDirect.
Exploring Camptothecin Derivatives from the Chinese Tree of Camptotheca acuminata as Breast Cancer Protease Inhibitors: Insights from Multi-Scale Computational Analysis
Abstract
Background
Breast cancer is a highly prevalent and lethal type of cancer that affects women worldwide.
Aims
This study aimed to explore active alkaloid-induced camptothecin (CPT) derivatives as efficacious agents for the treatment of triple-negative breast cancer (TNBC) by molecular docking simulation.
Methods
First of all, the DFT method from Material Studio 8.0 was executed to optimize ligands and to evaluate the quantum descriptors. The binding affinities of a series of twelve ligands with human topoisomerase IIα (5GWK) and p53 (4OQ3) were assessed. A protein data bank (PDB) was used to obtain the 3D structure of PDB ID: 5GWK (human topoisomerase II α in complex with DNA and etoposide) and PDB ID: 4OQ3 (Tetra-substituted imidazoles as a new class of inhibitors of the p53-MDM2 interaction). SwissADME and admetSAR - 2.0 were used to perform the absorption, distribution, metabolism, excretion, and toxicity (ADMET). Molecular dynamic simulations were conducted using the Desmond software suite.
Results
Ligand L05 emerged as a standout, demonstrating the highest binding affinity for both proteins, thereby positioning itself as a potential dual-targeting therapeutic agent. Notably, all ligands exhibited a propensity for higher binding affinity with 5GWK over p53. Pharmacokinetic profiling further delineated the drug-like attributes of the ligands, which included a molecular weight spectrum of 372.54 to 420.65 g/mol, rotatable bonds ranging from one to four, hydrogen bond acceptors between four to six, and hydrogen bond donors limited to zero or one, which satisfied the drug-likeness properties.
Conclusion
The comparative analysis of binding energies obtained from PyRx and Glide molecular docking simulations of twelve ligands with human topoisomerase IIα (5GWK) and p53 (4OQ3) provides insights into the efficacy of these computational tools in computer-aided drug discovery for TNBC.
1. INTRODUCTION
Breast cancer is a highly prevalent and lethal type of cancer that affects women worldwide. Triple-negative breast cancer (TNBC), among its various subtypes, presents unique challenges due to its aggressive nature and lack of targeted treatment options [1]. Despite the progress made in cancer therapy, TNBC is still associated with a poor prognosis and limited survival rates [2]. Consequently, there is a pressing need for innovative therapeutic approaches to enhance patient outcomes and decrease mortality rates [3].
TNBC is characterized by the absence of estrogen receptor (ER), progesterone receptor (PR), and human epidermal growth factor receptor 2 (HER2) expression, rendering it resistant to hormone-targeted therapies and HER2-directed treatments [4]. TNBC accounts for approximately 15-20% of all breast cancer cases and is linked to a younger age at diagnosis [5], higher tumor grade, elevated risk of metastasis, and a worse prognosis compared to other breast cancer subtypes [6]. Despite significant advancements in breast cancer treatment, TNBC remains a clinical challenge due to its heterogeneous nature and absence of specific molecular targets [7].
TNBC management heavily relies on conventional chemotherapy, which may result in systemic toxicity and restricted efficacy, especially for patients with advanced or metastatic disease [8]. Although conventional chemotherapy is somewhat effective, it often leads to systemic toxicity and drug resistance, highlighting the necessity for more selective and less toxic therapeutic agents [9].
Camptothecin (CPT), which is a natural alkaloid derived from the Chinese tree Camptotheca acuminata, has garnered significant attention due to its potent anticancer properties [10]. The cytotoxic effects of CPT are exerted through the inhibition of topoisomerase I (Topo I), a crucial enzyme involved in DNA replication and transcription [11]. By inducing DNA damage and interfering with the processes of DNA strand cleavage and resealing, CPT disrupts the proliferation and survival of cancer cells [12]. Despite its promising activity against various cancer types, including TNBC, the clinical utility of CPT has been restricted due to its poor solubility and considerable toxicity, particularly hematological and gastrointestinal adverse effects [13]. To overcome these limitations, researchers have explored the development of CPT derivatives with improved pharmacokinetic properties and enhanced anticancer efficacy [14].
In silico methodologies, such as molecular docking, absorption, distribution, metabolism, excretion, and toxicity (ADMET) prediction, as well as molecular dynamics simulations, have emerged as highly useful instruments in the realm of drug discovery and development [15, 16]. In silico approaches have been employed to repurpose approved drugs for TNBC treatment. A study utilized computational methods to identify existing drugs with potential efficacy against TNBC, focusing on their safety profiles and the feasibility of repurposing. Additionally, the study explored the use of gold nanoparticles to deliver these drugs, enhancing their therapeutic potential [17]. These computational methods enable scientists to anticipate the binding interactions between small molecules and target proteins [15], evaluate pharmacokinetic properties [16], and enhance drug design strategies in a manner that is both cost-effective and time-efficient [17]. Moreover, density functional theory (DFT), a quantum mechanical approach, offers valuable insights into the electronic structure and properties of molecules, thereby facilitating the comprehension of their chemical reactivity and stability. Through the modeling of molecular systems at the atomic level, DFT calculations provide significant information for comprehending the mechanisms of drug action and predicting the activity of novel compounds [18].
This study aimed to use in silico and DFT methods to explore the processes behind alkaloid-induced CPT and its derivatives in TNBC therapy. In silico approaches have been used to repurpose approved drugs for TNBC treatment, focusing on drug safety profiles and the potential of gold nanoparticles for enhanced delivery. Computational studies have also identified novel targets and inhibitors, such as compound 670551 for CLK2, and explored multitarget therapies like resveratrol. Additionally, pathway cross-talk inhibition analyses have led to the discovery of novel drug target pathways, offering new therapeutic strategies for TNBC [19]. More specifically, the main goals were to carry out molecular docking experiments in order to clarify the binding relationships between CPT derivatives modified by alkaloids and their molecular targets, such as Topo I and other pertinent proteins involved in the pathophysiology of TNBC, and to investigate the electronic structure and characteristics of the alkaloid-modified CPT derivatives using DFT simulations in order to shed light on their stability and chemical reactivity.
2. MATERIALS AND METHODS
2.1. Ethics Statement
This study did not necessitate ethics clearance, as it was classified as a molecular docking and in silico study.
2.2. Optimization and Ligand Preparation
Using sophisticated drawing tools or reliable databases, the precise 3D model of the ligand was meticulously crafted, taking into account atom types, charges, and bond orders by ChemDraw software. Subsequently, the ligands were optimized for molecular geometry carried out using a force field like UFF to ensure a valid starting point, meticulously checking for steric clashes and unrealistic bond parameters. The “Build Parameters” tool in Material Studio meticulously generated a detailed parameter file for the ligand, defining atom types, partial charges, and force field parameters essential for subsequent DFT calculations. Within the Material Studio environment, a new DFT calculation framework was established through the suitable method of B3LYP from the DMol3 code with a basis set like 6-31+G (d, p) hybrid DFT method tailored to specific research requirements [20]. The incorporation of solvent effects and other relevant calculation parameters was executed as needed. Subsequently, an input file encompassing the optimized geometry, chosen DFT settings, and the ligand's parameter was generated seamlessly. This comprehensive input file was then submitted for DFT calculations, with continuous monitoring of energy and geometry optimization progress. Upon convergence, the optimized geometry alongside the total energy was meticulously extracted, forming the foundation for robust computational analysis. A thorough examination of bond lengths, angles, and vibrational frequencies was conducted to derive profound insights into the ligand's structural dynamics and its interactions within the system. Utilizing the DFT functional, a multitude of quantum properties, including LUMO and HOMO energies, energy gap (E gap), ionization potential (I)(1), electron affinity (A)(2), electronegativity (χ) (3), electrophilicity (ω) (4), chemical potential (μ) (5), hardness (η) (6), and softness (s) (7), and were calculated using established equations (Eq. (1-7)) [21]. This rigorous computational approach ensures a comprehensive understanding of the ligand's behavior and its implications in various chemical processes using the following equations [21] Eq. (1-7):
![]() |
(1) |
![]() |
(2) |
![]() |
(3) |
![]() |
(4) |
![]() |
(5) |
![]() |
(6) |
![]() |
(7) |
2.3. Protein Preparation and Collection
The meticulous process of selecting and preparing the PDB ID: 5GWK (human topoisomerase II α in complex with DNA and etoposide) and PDB ID: 4OQ3 (Tetra-substituted imidazoles as a new class of inhibitors of the p53-MDM2 interaction), for molecular docking, was undertaken to ensure the reliability of the subsequent docking simulations [22, 23]. These proteins were found in different plant disease-causing fungi evaluated through the X-ray diffraction method with highly stable configurations listed in Table 1. These proteins were obtained from the protein data bank (PDB) (which can be accessed at https://www.rcsb.org/, on 10th March, 2024) [24].
The selection of these proteins was based on their biological relevance, specifically their potential association with the study objectives. In order to create a clean protein template for docking using Discovery Studio, co-crystallized ligands, water molecules, and ions were removed. Additionally, the protein structures underwent energy minimization to optimize their conformations and alleviate any steric clashes. This ensured that the selected fungal proteins were properly prepared for accurate and meaningful molecular docking investigations.
2.4. Molecular Docking and Visualization of Docking
2.4.1. Molecular Docking by PyRx
The molecular docking analysis was conducted using PyRx software by employing the AutoDockWizard option [25]. To evaluate the binding affinity between the ligand and individual macromolecules, essential parameters (Table 2) were obtained and utilized. In this process, the protein was loaded as a macromolecule, and the ligand was loaded separately. Subsequently, the loaded ligand underwent optimization for maximum energy, considering parameters, such as grid surface area, center of the grid, and grid dimensions. The goal was to ensure adequate coverage of the total surface area of both the ligand and the protein. The docking process was initiated with the specified parameters outlined in Table 2 within the PyRx software's AutoDock Wizard option. Following the docking procedure, the resulting ligand-protein docking complex was further analyzed for non-covalent interactions using Discovery Studio visualization [26].
2.4.2. Molecular Docking by Glide from Schrodinger Suite
In this research, glide docking was utilized as the molecular docking technique to examine the binding interactions between a variety of ligands and a target protein. The molecular docking was carried out using the Glide tool on the Schrodinger suite. For each protein structure, a grid box with an inner box was centered on the corresponding ligand (Table 2) [27]. There were, however, no boundaries, and the setting parameters were set to default. Following that, conformational sampling was put into effect for the ligands, and their anticipated binding affinities were then utilized to evaluate them. The Schrödinger software suite includes the Glide docking tool, which enables a systematic search of the conformational space to facilitate a comprehensive examination of ligand-protein interactions. Considerations like attachment modes, bonding by hydrogen patterns, and energetics were thoughtfully taken into account when analyzing the docking results. This method produced insightful information about the connections between structure and activity as well as possible binding locations that are essential for the logical design of novel ligands with enhanced pharmacological profiles. By defining the binding (active) site residues that were found, the binding site receptor grid for plant pathogenic fungal proteins was created. The docked conformers were evaluated using the Glide (G) score (Eq. 8) [28]. The G Score was calculated using equation Eq. (8), [28] which is as follows:
![]() |
(8) |
Wherein vdW denotes van der Waals energy, Coul denotes Coulomb energy, Lipo denotes lipophilic contact, HBond indicates hydrogen-bonding, Metal indicates metal-binding, BuryP indicates penalty for buried polar groups, RotB indicates penalty for freezing rotatable bonds, Site denotes polar interactions in the active site and a = 0.065 and b = 0.130 are coefficients of vdW and Coul, respectively [28].
2.5. Pharmacokinetics and ADMET Studies
The ADMET process is a critical component of assessing fungicide development, ensuring that potential candidates meet safety and efficacy standards before reaching the market. The procedure involves a comprehensive series of assessments to evaluate the pharmacokinetic and toxicological properties of the compounds [29, 30]. The ADMET criterion was obtained by use of the SwissADME and pkCSM online tools (http://biosig.unimelb.edu.au/pkcsm/prediction_single/adme_1643650057.59; accessed from the October 10th, 2023) [31]. Throughout the ADMET process, a combination of experimental techniques, such as in vitro assays, animal studies, and computational modeling, was employed to gather comprehensive data on the compound's pharmacokinetic and toxicological properties. The results of these assessments informed fungicide development, guiding compound optimization, formulation, and regulatory approval processes.
2.6. Lipinski’s Rule and Pharmacokinetics
SwissADME, accessed on October 9th, 2023, was utilized to predict pharmacokinetics and assess fungicide-likeness metrics. This online database, available at http://www.swissadme.ch/index.php [32], is significant in terms of its important and adaptable functions that make information easier to access. To clarify the fungicide-likeness characteristics of ligands, a number of pharmacokinetic parameters were calculated, including molecular weight, bond rotation numbers (NRB), lipophilicity, hydrogen bond donors (HBD), and hydrogen bond acceptors (HBA).
2.7. Molecular Dynamics
Simulations were conducted using the Desmond software suite (Schrödinger Release 2024-1: Desmond Molecular Dynamics System, D. E. Shaw Research, New York, NY, 2024) in accordance with established molecular dynamics (MD) protocols [33]. The molecular system, which consisted of a biologically relevant complex, was prepared using the OPLS-AA force field, and the solvent environment was described using an appropriate water model. To ensure solvent relaxation, the system underwent a staged equilibration process that involved restrained dynamics on the solute. Following this, MD runs were carried out under NPT conditions, with a finite time step (100 ns) and temperature control. Desmond outputs trajectory files, energy logs, and other data that can be further analyzed or visualized using external tools. Trajectory analysis, performed using Maestro and supplemented by external tools, included measurements, such as root mean square deviation (RMSD), root mean square fluctuation (RMSF), radius of gyration, and energy profiles. Visualization tools were used to examine conformational changes and intermolecular interactions throughout the simulation [33]. This comprehensive computational investigation followed established best practices and parameters, providing a thorough exploration of the dynamic behavior of the molecular system under investigation.
Protein Name with PDB ID | Grid Box Size (PYRX) | Grid Box Size (Glide) | ||
---|---|---|---|---|
Center | Dimension (Å) | Center | Dimension (Å) | |
Homo sapiens (PDB 5GWK) |
X = 26.38 | X = 111.29 | X =40 | X =30 |
Y = -31.51 | Y= 92.68 | Y =-30 | Y =30 | |
Z = -44.07 | Z = 106.96 | Z =-50 | Z =30 | |
Homo sapiens (PDB 4OQ3) |
X = 12.01 | X = 32.21 | X =10 | X =20 |
Y = -19.35 | Y = 36.91 | Y =-15 | Y =20 | |
Z = 15.39 | Z = 36.41 | Z =15 | Z =30 |
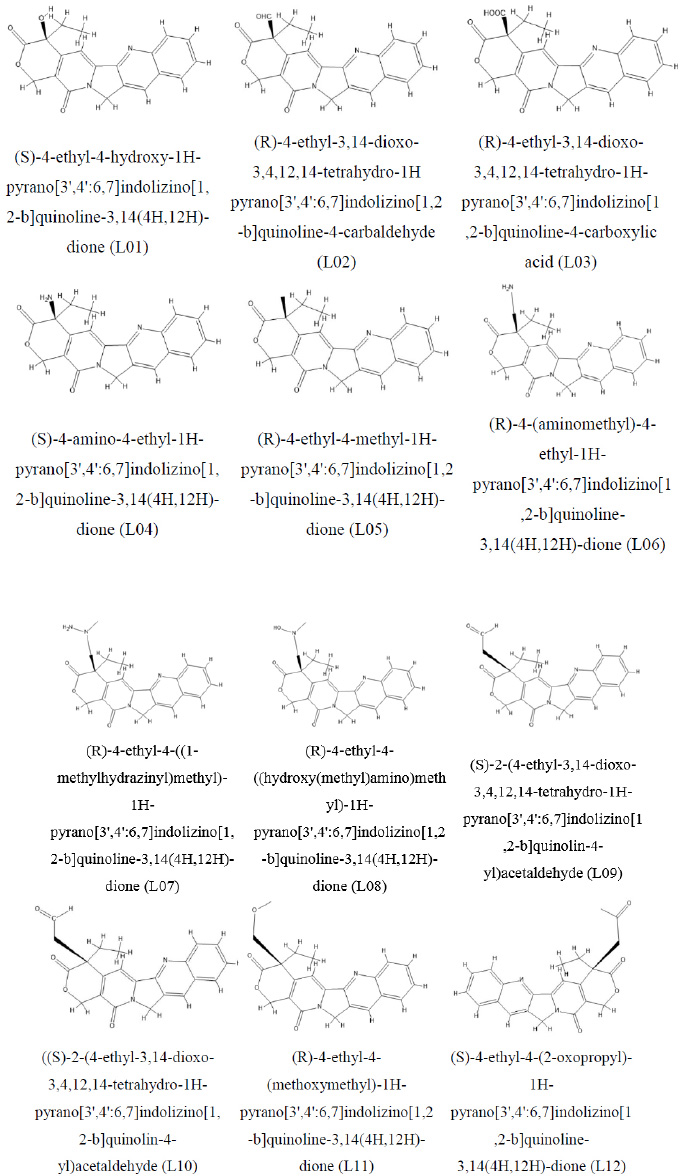
Molecular structure of camptothecin and its derivative.
3. RESULTS AND DISCUSSION
3.1. DFT Optimization and Analysis
3.1.1. Chemistry and Optimized Chemical Structures
Camptothecin derivatives stem from the Chinese tree Camptotheca acuminata, referred to as the “happy tree” or “cancer tree.” Camptothecin, a natural alkaloid found in the tree's bark and stem, has captivated medicinal chemistry with its potent anticancer potential. Particularly, it disrupts the enzyme topoisomerase I, which is pivotal in DNA replication and transcription. Camptothecin derivatives have undergone extensive scrutiny and refinement to heighten their pharmacological attributes, encompassing solubility, stability, and specificity, along with increased anticancer efficacy. At a molecular level, camptothecin derivatives possess a pentacyclic quinoline or indole structure, integral for their interaction with topoisomerase I. Strategic modifications are made at various sites along the camptothecin scaffold, enhancing its pharmaceutical properties. Key alterations involve introducing varied substituents at C-7, C-9, and C-10 positions alongside modifications to the lactone ring at C-20. These molecular modifications influence the compound's stability, solubility, and potency, as depicted in its chemical structure in Fig. (1).
Camptothecin derivatives emerge as promising contenders in cancer therapy, especially against solid tumors like colorectal, ovarian, lung, and pancreatic cancers. Notably, derivatives like irinotecan and topotecan have gained regulatory approval in several nations for clinical use. These analogs exhibit improved pharmacokinetics and reduced toxicity compared to camptothecin. Ongoing research in camptothecin derivatives aims to develop new analogs with enhanced anticancer activity and reduced side effects. Methodologies for optimization span structure-activity relationship exploration, prodrug innovation, nanoparticle formulations, and synergistic coupling with other anticancer agents. Collectively, camptothecin derivatives stand as a vanguard in the anticancer arsenal, with continuous exploration aimed at elevating their efficacy and clinical applicability.
3.1.2. Optimized Structure of Tested Compounds
In the quest to harness computational techniques for quantum calculations of chemical entities, optimizing molecular arrangement becomes paramount to achieving accurate structural geometry [34]. This investigation prioritized identifying the most stable configuration of each chemical structure to refine computational parameters efficiently. Employing DFT, all compounds underwent computational refinement, resulting in the observation of their principal and most stable configurations with minimal energy expenditure. The ligands are illustrated in Fig. (2).
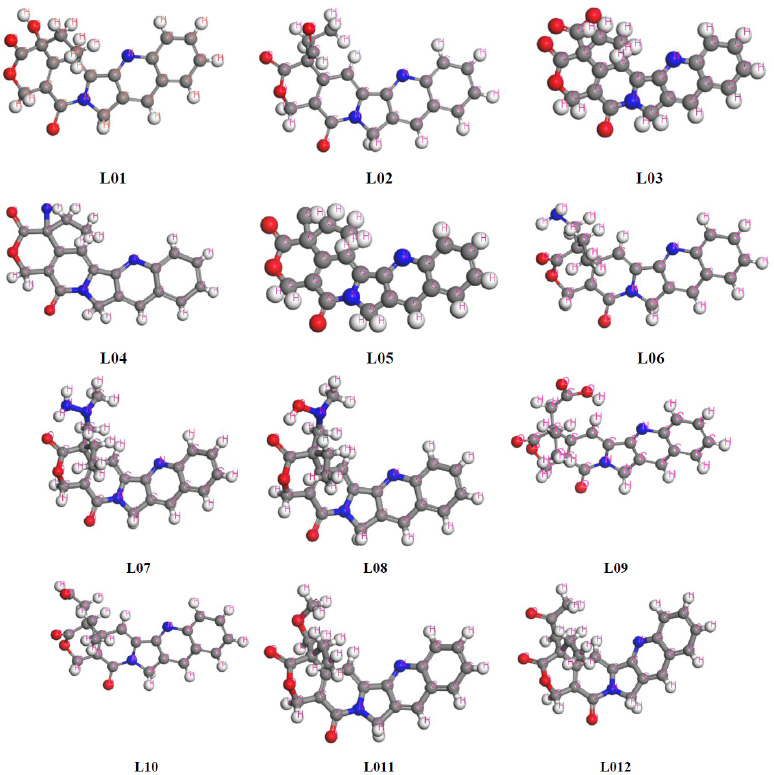
Optimized chemical structure of ligands.
3.2. HOMO, LUMO, and Chemical Reactivity Descriptors
The calculations of the molecular parameters, including LUMO, HOMO, energy gap (ΔE gap), chemical potential (µ), electronegativity (χ), hardness (η), softness (σ), and electrophilicity (ω), were conducted using the DFT, and the results are presented in Table 3. The HOMO-LUMO energy gap serves as a crucial indicator of chemical reactivity and stability in molecules. In this context, a wider gap suggests higher chemical stability, while a narrower gap implies increased reactivity. The findings reveal that the HOMO–LUMO gaps range from 2.474 eV to 10.040 eV across all studied ligands. Notably, ligands L16 exhibit minor energy gaps and minimal softness values, indicating their potential for reactivity and reduced stability. In contrast, L05 stands out with the greatest hardness and the largest energy gap, emphasizing its stability. It has been observed that the order of the energy gap is L05<L06< L04<L03< L01<L09< L10<L11<L12< L02<L08<L07. Table 3 further illustrates that the softness values are approximately 0.119 or less than 0.30, underscoring the potential for faster degradation and disintegration for elements with higher softness values [35-38]. Conversely, hardness, a crucial stability indicator, is reflected in the compounds' resistance to changes in electron configuration [39-41]. Higher hardness values signify increased stability and resistance to changes, providing valuable insights into the chemical behavior of these compounds.
Ligands | LUMO | HOMO | A = -LUMO | I = -HOMO | Energy gap = I-A | Chemical Potential (µ)= -I+A/2 | Hardness (η)= I-A/2 | Electronegativity (x)=I+A/2 | Softness (σ)=1/n | Electrophilicity (ω)=µ2/2η |
---|---|---|---|---|---|---|---|---|---|---|
L01 | -9.02 | -1.85 | 9.02 | 1.85 | -7.170 | -5.443 | -3.585 | 5.443 | -0.278 | -4.131 |
L02 | -9.15 | -2.03 | 9.15 | 2.03 | -7.110 | -5.594 | -3.557 | 5.594 | -0.281 | -4.398 |
L03 | -8.04 | -1.10 | 8.04 | 1.10 | -6.936 | -4.576 | -3.468 | 4.576 | -0.288 | -3.018 |
L04 | -8.19 | -1.34 | 8.19 | 1.34 | -6.855 | -4.767 | -3.427 | 4.767 | -0.291 | -3.315 |
L05 | -8.61 | -1.69 | -0.04 | 8.94 | 8.986 | -4.450 | 4.493 | 4.450 | 0.222 | 2.203 |
L06 | -9.09 | -2.51 | 9.09 | 2.51 | -6.581 | -5.805 | -3.290 | 5.805 | -0.303 | -5.121 |
L07 | -8.19 | -1.85 | 8.19 | 1.85 | -6.338 | -5.025 | -3.169 | 5.025 | -0.315 | -3.984 |
L08 | -8.81 | -1.99 | 8.81 | 1.99 | -6.819 | -5.404 | -3.409 | 5.404 | -0.293 | -4.283 |
L09 | -9.03 | -1.87 | 9.03 | 1.87 | -7.156 | -5.454 | -3.578 | 5.454 | -0.279 | -4.156 |
L10 | -9.12 | -1.96 | 9.12 | 1.96 | -7.166 | -5.543 | -3.583 | 5.543 | -0.279 | -4.287 |
L11 | -9.02 | -1.90 | 9.02 | 1.90 | -7.124 | -5.462 | -3.562 | 5.462 | -0.280 | -4.187 |
L12 | -9.02 | -1.90 | 9.02 | 1.90 | -7.124 | -5.462 | -3.562 | 5.462 | -0.280 | -4.187 |
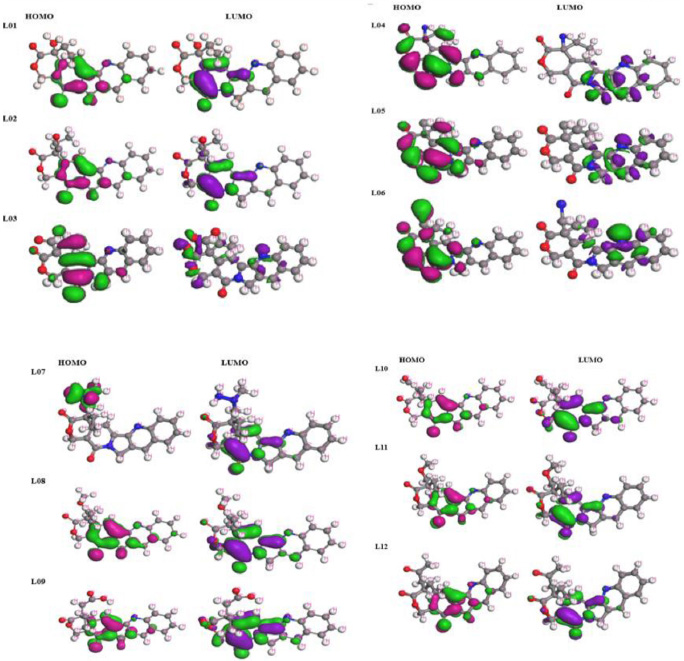
Frontier molecular orbitals diagram for HOMO and LUMO.
Table 3 presents a comprehensive analysis of the electronic properties of twelve ligands (L01-L12), offering insights into their potential reactivity. Ligands L05 and L06 stand out with high LUMO energies, indicating a propensity for electron acceptance, while L02 and L06 display lower HOMO energies, suggesting resistance to electron donation. Smaller energy gaps for ligands L03 and L04 imply higher reactivity. Chemical potential varies notably among ligands, with L05 exhibiting a high tendency to gain electrons and L02 favoring electron loss. Ligands L05 and L06 also demonstrate lower hardness and higher softness, indicating susceptibility to electron cloud distortion and ease of electron donation. Despite a high chemical potential, ligand L06 shows higher electrophilicity due to its significantly lower hardness. However, the evidence suggests that ligands with smaller energy gaps and lower hardness, such as L03, L04, L09, and L07, will be more reactive in electron transfer activities. While ligand L05 differs in certain ways (such as having a higher energy gap and being softer), it appears to be a highly reactive ligand with potential uses in catalysis and drug creation. The overall trend in this data indicates that the electrical characteristics of these ligands could be fine-tuned for certain applications in chemical processes, depending on whether they require electron donation or acceptance.
3.3. Frontier Molecular Orbital (FMO): HOMO and LUMO
Through FMO analysis, we delved into the dynamics, uncovering the precise locales primed for protein folding, thereby highlighting the active pharmacophores or functional groups. The highest value of HOMO, a linchpin in myriad chemical reactions, especially electron transfers, stands as a crucial player [42-46]. Not only does the HOMO serve as an electron donor, but it also assumes a pivotal role in nucleophilic attacks by relinquishing its electron density. Conversely, the LUMO, characterized by its electron-free state, interacts with electrons from the HOMO of another molecule during reactions. In scenarios demanding electrophilic attacks, where it beckons electrons, the LUMO steps in as the electron acceptor. Both the LUMO and HOMO orbitals are delineated with a dark blue hue for their positive terminal and a pink hue for their negative node. A narrower energy gap paves the way for fungicides to seamlessly integrate with proteins. Fig. (3) unravels an array of molecular territories corresponding to HOMO and LUMO, providing invaluable insights into their reactivity and potential pharmacological activity.
3.4. Electrostatic Potential Map for FMO
The significance of comprehending the reactivity and stability of molecules in chemical reactions lies in the electrostatic potential map for FMO. The role of the HOMO and the LUMO, known as the FMOs, in determining a molecule's reactivity is crucial, as suggested by FMO theory. Analyzing the HOMO electrostatic potential map provides valuable insights into areas of electron density that are most susceptible to nucleophilic attacks. Similarly, the LUMO map identifies regions of electron deficiency, indicating sites that are prone to electrophilic attacks. By studying these maps, chemists are able to predict the mechanisms and outcomes of chemical reactions.
Furthermore, comparing the electrostatic potential maps of different molecules allows for the evaluation of their relative reactivity and selectivity in various reactions. Understanding the distribution of electrostatic potential in FMOs is, therefore, a fundamental aspect in rationalizing and predicting chemical reactivity, as well as designing new molecules with specific properties. As shown in Fig. (4), it can be observed that the values of negative (from -8.22 to -3.490) and positive ends (from about 4.50 to 2.00) differ. The reactivity and selectivity of the optimized molecules are not constant, and there is no specific binding mechanism that explains the binding affinities for proteins. Additionally, it is noted that the positive charge of the molecules is lower than their negative charge.
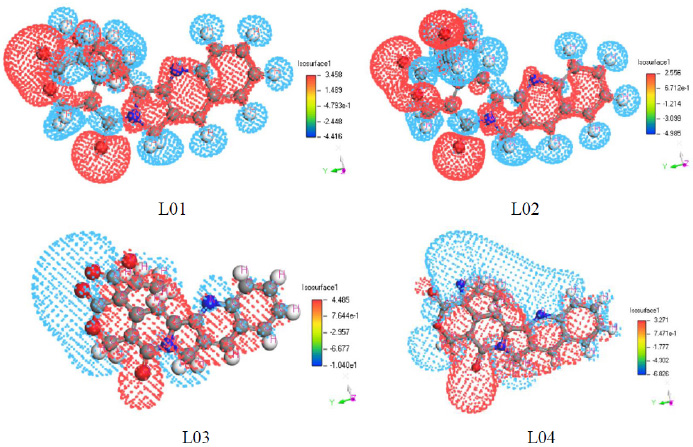
Electrostatic potential map for frontier molecular orbital of L01-L04.
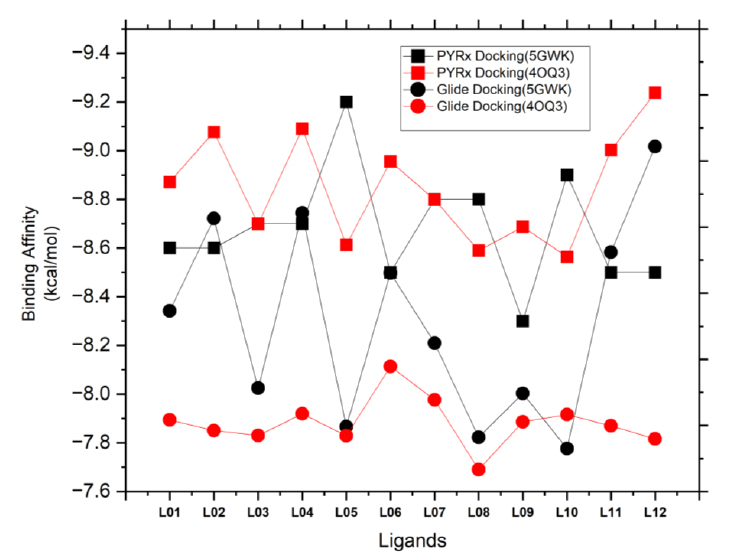
A comparative study of molecular docking using AutoDock in PyRx and glide in the schrödinger suite.
3.5. Molecular Docking Analysis
3.5.1. Result of Auto Docking by PyRx
Molecular docking simulations were conducted to authenticate the pharmacological findings. The binding affinities of twelve ligands with two distinct targets, human topoisomerase IIα (5GWK) and the p53 (4OQ3), were investigated using Auto docking by PyRx simulations (Fig. 5). The interaction between protein and ligand is crucial for the development of structurally oriented fungicides. It is widely accepted that docking scores higher than -6.00 kcal/mol indicate a standard fungicide [47, 48]. Additionally, molecular docking is a reliable approach for understanding the engagement of two molecules and identifying the optimal configuration for ligand binding. Through in-silico experiments, it was revealed that the fungicide compounds in Table 4 exhibit excellent binding affinity to the target proteins.
The binding affinities, hydrogen bond (H-bond) interactions, and hydrophobic interactions of the ligands with 5GWK and 4OQ3 provide key insights into their molecular behavior. L05 exhibits the strongest binding affinity (-9.2 kcal/mol for 5GWK), indicating its potential as a potent binder (Fig. 5). Hydrogen bonding is generally low, with L01, L03, and L08 having 3-4 H-bonds, suggesting moderate electrostatic interactions. Hydrophobic interactions are prominent, especially in L02, L05, and L07, with up to 10 hydrophobic bonds, highlighting their non-polar binding nature. These results indicate that hydrophobic interactions are crucial for stabilizing ligand-target binding, guiding further optimization for specific applications. However, ligand L05 showed the highest affinity for both targets, highlighting its potential as a dual-target agent. L06, however, had weaker binding, emphasizing the role of molecular structure in ligand-protein interactions. Variations in binding profiles between the two targets revealed distinct molecular mechanisms, offering insights into designing effective anticancer agents for triple-negative breast cancer.
3.5.2. Result of Molecular Docking by Glide from Schrodinger Suite
The binding affinities of twelve ligands with two triple-negative breast cancer proteins, human topoisomerase IIα (5GWK) and p53 (4OQ3), were investigated via glide docking simulations. The ligand preparation product, Ligprep, from the Schrodinger suite, was utilized for the purpose of generating high-quality 2D structures at the atomic level. The process of ligand preparation involved various steps, such as 2D-3D conversions, generation of structural variations, correction, verification, and optimization. In order to generate the receptor grid, the receptor grid generation module of the Glide applications [49-51] within Maestro (Schrödinger Release 2024-1: Glide, Schrödinger, LLC, New York, NY, 2024.) was employed. Conducting in silico experiments, it was discovered that the fungicides listed in Table 5 possess remarkable binding affinity towards the target proteins 5GWK and 4OQ3, highlighting significant variations in ligand-protein interactions, with values ranging from -6.166 to -9.017 kcal/mol (Fig. 5). Overall, the ligands demonstrated strong binding affinities, with L12 showing the highest affinity for 5GWK (-9.017 kcal/mol), indicating its potential as a potent binder. In contrast, L05 exhibited the weakest binding affinity for 5GWK (-7.866 kcal/mol) (Fig. 5), underlining the role of ligand structure in modulating binding strength. Across both targets, hydrophobic interactions were found to be predominant, with ligands like L05, L08, and L12 exhibiting a higher number of hydrophobic bonds (up to 12), suggesting their strong non-polar interaction tendencies. Ligands, such as L03 and L06, showed relatively more hydrogen bonding, reflecting the diversity of electrostatic interactions within the binding sites. Interestingly, binding affinities for both targets varied, with the 4OQ3 target generally showing slightly weaker binding compared to 5GWK, emphasizing the target-specific nature of ligand binding. These findings highlight the complexity of ligand-target interactions and offer valuable insights into optimizing ligand design, particularly for applications targeting cancer therapies.
Studied Ligands | 5GWK | 4OQ3 | ||||
---|---|---|---|---|---|---|
Binding Affinity (kcal/mol) | No. of H Bond | No. Hydrophobic Bond | Binding affinity (kcal/mol) | No. of H Bond | No. Hydrophobic Bond | |
L01 | -8.6 | 03 | 02 | -8.3 | 02 | 05 |
L02 | -8.6 | 01 | 06 | -7.8 | 00 | 09 |
L03 | -8.7 | 03 | 08 | -7.9 | 02 | 04 |
L04 | -8.7 | 01 | 06 | -7.9 | 00 | 08 |
L05 | -9.2 | 01 | 09 | -8.1 | 00 | 06 |
L06 | -8.5 | 02 | 06 | -7.5 | 00 | 04 |
L07 | -8.8 | 01 | 09 | -7.6 | 00 | 05 |
L08 | -8.8 | 04 | 06 | -7.8 | 00 | 04 |
L09 | -8.3 | 02 | 06 | -7.7 | 01 | 10 |
L10 | -8.9 | 01 | 07 | -7.5 | 01 | 10 |
L11 | -8.5 | 00 | 07 | -7.5 | 00 | 09 |
L12 | -8.5 | 00 | 06 | -8.2 | 00 | 03 |
Studied Ligands | 5GWK | 4OQ3 | ||||
---|---|---|---|---|---|---|
Binding Affinity (kcal/mol) | No. of H Bond | No. Hydrophobic Bond | Binding Affinity (kcal/mol) | No. of H Bond |
No. Hydrophobic Bond |
|
L01 | -8.342 | 02 | 07 | -6.542 | 02 | 10 |
L02 | -8.721 | 01 | 07 | -6.461 | 01 | 10 |
L03 | -8.024 | 04 | 03 | -6.423 | 01 | 10 |
L04 | -8.744 | 01 | 09 | -6.587 | 03 | 09 |
L05 | -7.866 | 01 | 07 | -6.421 | 01 | 12 |
L06 | -8.498 | 03 | 05 | -6.947 | 02 | 10 |
L07 | -8.209 | 03 | 09 | -6.692 | 02 | 09 |
L08 | -7.822 | 02 | 07 | -6.166 | 01 | 12 |
L09 | -8.003 | 02 | 08 | -6.526 | 02 | 11 |
L10 | -7.776 | 01 | 07 | -6.581 | 02 | 09 |
L11 | -8.583 | 02 | 07 | -6.496 | 01 | 12 |
L12 | -9.017 | 03 | 05 | -6.397 | 01 | 10 |
3.6. A Comparative Study for Docking Results
The comparative analysis of binding energies obtained from PyRx and Glide molecular docking simulations of twelve ligands with human topoisomerase IIα (5GWK) and p53 (4OQ3) provides insights into the efficacy of these computational tools in computer-aided drug discovery for triple-negative breast cancer. Overall, both methods demonstrated similar trends in ligand affinities for both protein targets, with notable variations in absolute values. Ligands consistently exhibited higher binding affinities with 5GWK compared to p53 across both docking platforms. However, there were discrepancies in binding energies between PyRx and Glide docking for some ligands, suggesting differences in their scoring functions and algorithms. The reasonable analysis of binding affinities from Glide docking (Table 5) and AutoDock from PyRx (Table 4) revealed consistent trends in ligand binding against 5GWK and 4OQ3 proteins for triple-negative breast cancer, with some notable differences between the two methods. Glide docking identified L12 as the strongest binder for 5GWK (-9.017 kcal/mol) and L01 for 4OQ3 (-6.542 kcal/mol), while AutoDock highlighted L05 as the best binder for 5GWK (-9.2 kcal/mol) and L01 for 4OQ3 (-8.6 kcal/mol). Despite the slight variation in binding scores, both methods identify L05 as a strong binder for 5GWK, with differences in rankings for other ligands, particularly for 4OQ3. Hydrogen bond interactions were found to be similar across both methods, ranging from 0 to 4, with L03 and L06 showing the highest number of H-bonds in Glide and L01 and L03 in AutoDock. Hydrophobic interactions are consistently significant, with L05 showing the highest number of hydrophobic bonds in both methods (12 for 4OQ3), underlining its strong hydrophobic characteristics. Both methods also revealed target-specific binding trends, with Glide suggesting stronger binding to 5GWK for most ligands and AutoDock showing similar patterns, further emphasizing the importance of considering these variations in the rational design of dual-target anticancer agents.
3.7. Protein-ligand Interaction for PyRx in View of Superpose/position
Aligning ligands post-docking is a crucial step in molecular docking studies, offering deep insights into ligand-protein interactions, as depicted in Fig. (6). This process involves comparing multiple ligand structures to evaluate their spatial alignment and binding orientations relative to a reference ligand or protein active site. By analyzing consistency in binding poses across various ligands, researchers can pinpoint common binding mechanisms and potential hotspots on the protein surface. Additionally, this analysis highlights unique interactions and conformational variations among ligands, providing valuable insights into the molecular determinants of binding affinity and specificity. Understanding induced fit and structural changes in the protein upon ligand binding facilitates the design of ligands with enhanced properties. Overall, ligand superposition after docking offers a comprehensive assessment of ligand-protein interactions, effectively guiding drug discovery efforts.
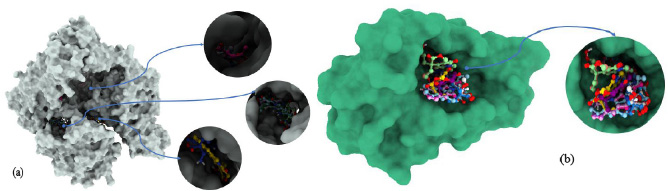
Molecular docking poses of (a): 5GWK and (b): 4OQ3.
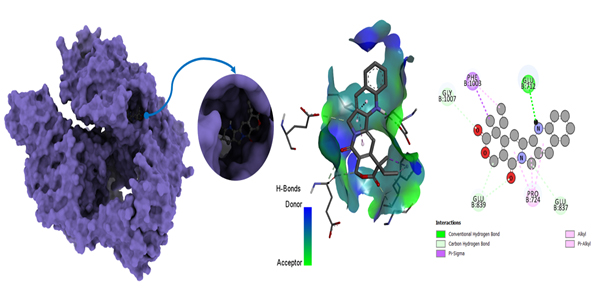
Molecular docking poses of triple-negative breast cancer PDB (5GWK)- L05.
The interactions of ligands with amino acid residues in the 5GWK protein provide essential insights into their binding mechanisms. Hydrogen bonds, with distances ranging from 1.54 to 2.72 Å, indicate strong electrostatic interactions, contributing to the stability of the ligand-protein complex. For instance, ligand L01 forms hydrogen bonds with GLY B:617 and SER B:621, while L05 interacts with GLU B:712 and PHE B:1003. Hydrophobic interactions, with distances from 3.14 to 5.47 Å, further stabilize these complexes. Ligands, such as L07 and L12, exhibit significant hydrophobic interactions, contributing to overall binding affinity and specificity.
Van der Waals interactions, though not explicitly detailed, also play a crucial role in stabilizing the binding, with distances ranging from 3.14 to 5.47 Å, suggesting favorable binding modes. Ligands like L04 and L06 show close interactions with residues, such as PHE A:807 and GLU B:712, enhancing binding stability. Additionally, ligands, such as L02 and L12, with fewer hydrogen bonds, rely on strong hydrophobic interactions for binding stability.
In summary, the combination of hydrogen bonding, hydrophobic interactions, and Van der Waals forces contributes to the strong binding of ligands to the 5GWK protein. Ligands like L12, L05, and L07, which exhibit consistent and strong interactions, show promise for further development as therapeutic agents for triple-negative breast cancer, providing valuable insights into drug design targeting the 5GWK protein.
3.8. Different Poses of Docking for Protein-ligand Interaction
3.8.1. View of PyRx Docking Poses
The identification of the ligand binding site with the receptor was carried out using Discovery Studio version 2020, as represented in Figs. 7-10. Moreover, their interaction is explained in Tables 6 and Table 7. Auto-docking was performed to identify the active site and determine amino acid residues, with hydrogen and hydrophobic bonds playing pivotal roles in docking score variation. Post-docking analyses were conducted to assess drug-protein pocket interactions, often comparing results with experimental data or crystallographic structures for validation. Scoring function assessment ensured accurate prediction of binding affinities, with room for improvement to enhance future projections' accuracy. Overall, the docking procedure, coupled with post-docking analyses, provides valid insights into protein-drug interactions. According to the results of PyRx docking, the ligand L05 had the most binding sides, with an H-bond count of one and five hydrophobic bonds, and L10 showed an H-bond count of two and seven hydrophobic bonds against the 5GWK. In the case of 4OQ3, ligand L01 had the most binding sides, with an H-bond count of two and five hydrophobic bonds, and L10 showed an H-bond count of zero and four hydrophobic bonds against the 4OQ3.
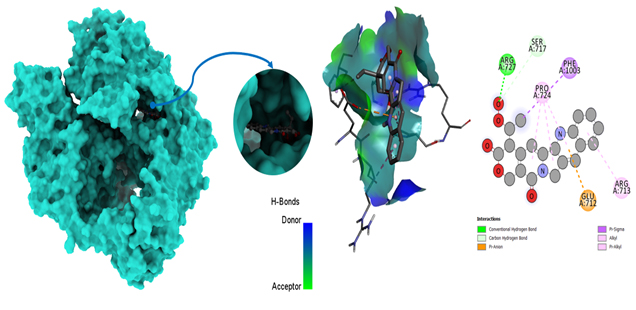
Molecular docking poses of triple-negative breast cancer PDB (5GWK) -L10.
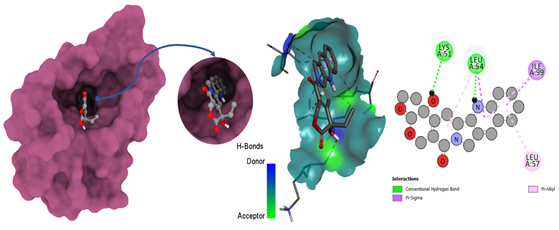
Molecular docking poses of triple-negative breast cancer PDB (4OQ3)-L01.
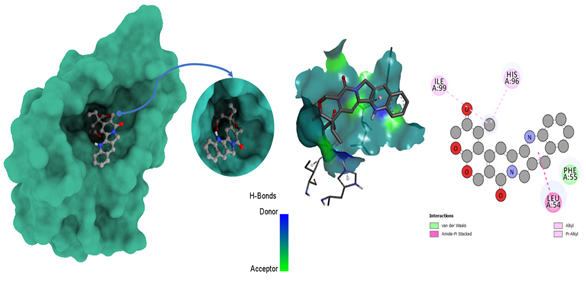
Molecular docking poses of triple-negative breast cancer PDB (4OQ3)- L12.
Ligands Interaction with Amino acid Residues and their Bond Distance (PDB ID 5GWK) | ||||||
---|---|---|---|---|---|---|
Active Sites | ||||||
Ligand No. | Hydrogen Bond | Hydrophobic Bond | Van Der Waals Bond | |||
The Interacting Residue of Amino Acid | Distance, Ao | The Interacting Residue of Amino Acid | Distance, Ao | |||
L01 | GLY B:617 GLY B:617 SER B:621 |
2.32 2.28 2.25 |
PHE A:807 PHE A:807 |
4.81 5.27 |
Absent | |
L02 | GLU A:71 | 2.30 | GLU A:837 PRO A:724 PRO A:724 PRO A:724 PHE A:1003 GLY A:1007 |
3.72 4.53 4.22 4.75 3.69 4.76 |
Absent | |
L03 | HIS A:759 LYS A:728 SER A:709 |
2.24 2.98 1.99 |
PRO A:724 PRO A:724 PRO A:724 PRO A:724 PHE A:1003 GLU A:837 ARG A:713 HIS A:759 |
4.15 4.28 4.34 4.42 4.98 3.69 4.29 5.26 |
Absent | |
L04 | LYS A:611 | 2.52 | LYS A:611 TYR B:805 TYR B:805 ALA B:801 LYS A:614 LYS A:614 |
1.78 4.63 4.99 4.82 3.60 4.93 |
Absent | |
L05 | GLU B:712 | 2.37 | PHE B:1003 PHE B:1003 GLY B:1007 GLU B:839 GLU B:839 PRO B:724 PRO B:724 PRO B:724 GLU B:837 |
3.43 4.39 3.41 3.14 3.70 4.53 4.13 4.65 3.75 |
Absent | |
L06 | LYS B:611 THR B:618N |
2.72 2.69 |
LYS B:611 ALA A:801 TYR A:805 PHE A:807 PHE A:807 ILE A:787 |
1.54 4.85 3.86 5.26 4.31 5.26 |
Absent | |
L07 | GLU B:839 | 3.07 | GLY B:1007 PHE B:1003 ILE B:715 LEU B:722 ARG B:713 GLU B:712 PRO B:724 PRO B:724 PRO B:724 |
3.79 4.70 3.52 3.57 5.24 3.89 4.94 5.31 5.41 |
Absent | |
L08 | GLU B:712 GLU B:712 HIS B:759 LYS B:728 |
2.99 2.11 2.14 2.89 |
HIS B:759 ARG B:713 PRO B:724 PRO B:724 PRO B:724 PHE B:1003 |
4.61 4.35 4.74 4.59 4.47 4.99 |
Absent | |
L09 | LYS B:728 GLU B:712 |
2.85 2.83 |
ARG B:713 HIS B:759 PRO B:724 PRO B:724 GLU B:837 PHE B:1003 |
3.96 4.77 4.83 4.35 3.55 4.98 |
Absent | |
L10 | ARG A:727 | 1.82 | SER A:717 PHE A:1003 PRO A:724 PRO A:724 PRO A:724 GLU A:712 ARG A:713 |
3.72 3.84 5.24 5.35 4.85 4.14 5.47 |
Absent | |
L11 | TYR B:805 TYR B:805 ALA B:801 LYS A:614 PHE B:807 PHE B:807 ILE B:787 |
4.51 5.51 5.47 5.08 5.15 4.20 5.06 |
Absent | |||
L12 | LYS A:614 LYS A:614 TYR B:805 TYR B:805 PHE B:807 GLY B:788 |
5.34 3.43 3.74 4.72 5.32 3.65 |
3.9. Protein-ligand Interaction from glide docking
Glide, developed by Schrödinger, is a widely used molecular docking program for predicting how small molecules (ligands) bind to target proteins. To analyze protein-ligand interactions from Glide docking, one must examine docking results and visualize binding modes. Glide provides docking scores, which estimate binding affinity; lower scores suggest stronger binding. Post-docking steps and analyses are crucial for comprehending and evaluating results. The software generates multiple binding poses (hypothetical configurations) for the ligand within the protein pocket; each assigned a score representing expected binding energy. Typically, lower scores indicate more favorable binding. Evaluation of the scoring function ensures accurate prediction of known binding affinities, with potential for future improvements to enhance accuracy. As mentioned in Table 5, according to the results of Glide docking, where the ligand L05 had the most binding sides, with an H-bond count of one and five hydrophobic bonds, and L10 showed an H-bond count of two and seven hydrophobic bonds against the 5GWK. In the case of 4OQ3, ligand L01 had the most binding sides, with an H-bond count of two and five hydrophobic bonds, and L10 showed an H-bond count of zero and four hydrophobic bonds against the 4OQ3, as shown in Fig. (11).
Ligands Interaction with Amino Acid Residues and their Bond Distance (PDB ID: 4OQ3) | ||||||
---|---|---|---|---|---|---|
Active Sites | ||||||
Ligand No. | Hydrogen Bond | Hydrophobic Bond | Van Der Waals Bond | |||
The Interacting Residue of Amino Acid | Distance, Ao | The Interacting Residue of Amino Acid | Distance, Ao | |||
L01 | LYS A:51 LEU A:54 |
2.54 2.23 |
LEU A:54 LEU A:54 ILE A:99 ILE A:99 LEU A:57 |
5.01 3.97 3.98 5.44 5.11 |
Absent | |
L02 | PHE A:55 LEU A:54 LEU A:54 HIS A:96 HIS A:96 ILE A:99 ILE A:99 VAL A:93 GLY A:58 |
5.14 3.44 4.16 5.07 4.89 5.07 5.19 4.87 3.42 |
Absent | |||
L03 | LEU A:54 LYS A:51 |
5.54 1.94 |
PHE A:55 ILE A:99 LEU A:54 LEU A:57 |
5.08 3.95 5.39 4.99 |
Absent | |
L04 | HIS A:96 HIS A:96 LEU A:54 LEU A:54 ILE A:99 ILE A:99 VAL A:93 GLY A:58 |
4.16 4.85 4.88 3.96 5.28 5.15 4.85 3.41 |
Absent | |||
L05 | ILE A:99 ILE A:99 HIS A:96 LEU A:54 LEU A:54 LEU A:54 |
4.34 4.50 3.99 4.46 5.25 4.04 |
Present | |||
L06 | HIS A:96 ILE A:99 LEU A:54 LEU A:54 |
4.06 4.44 4.08 5.37 |
Present | |||
L07 | VAL A:93 GLN A:59 LEU A:54 LEU A:54 LYS A:51 |
3.81 3.75 4.25 4.67 5.31 |
Present | |||
L08 | ILE A:99 HIS A:96 LEU A:54 LEU A:54 |
4.43 4.07 5.33 4.20 |
Present | |||
L09 | GLN A:24 | 2.37 | LYS A:51 LEU A:54 HIS A:96 HIS A:96 HIS A:96 VAL A:93 VAL A:93 VAL A:93 ILE A:99 ILE A:99 |
4.49 4.63 2.04 4.08 4.67 5.39 4.99 5.38 5.15 4.21 |
Absent | |
L10 | GLN A:24 | 2.58 | LEU A:54 LEU A:54 LEU A:54 HIS A:96 HIS A:96 ILE A:99 ILE A:99 LEU A:57 VAL A:93 VAL A:93 |
4.43 4.57 5.38 4.64 4.86 3.77 4.76 5.35 5.11 5.29 |
Absent | |
L11 | LEU A:54 LEU A:54 HIS A:96 HIS A:96 ILE A:99 ILE A:96 VAL A:93 GLY A:58 PHE A:55 |
3.45 4.82 4.16 4.86 5.14 5.14 4.76 3.48 5.43 |
Absent | |||
L12 | ILE A:99 HIS A:96 LEU A:54 |
5.24 4.12 4.17 |
Present |
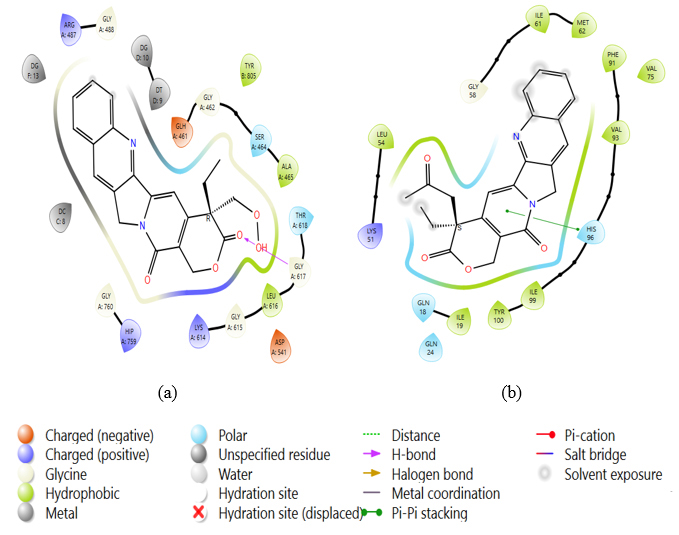
2D stick diagrams of (a) PDB: 5GWK-L04 and (b) PDB: 4OQ3-L12, illustrating hydrogen bonds and pi-pi stacking formed with the amino-acid residues at the binding pocket of triple-negative breast cancer.
Ligand No. | Molecular Weight, g/mol | Number of Rotatable Bonds | Hydrogen bond acceptor | Hydrogen Bond Donor | Topological Polar Surface Area (Å2) | Lipinski’s Rule | Bioavailability Score | |
---|---|---|---|---|---|---|---|---|
Result | Violation | |||||||
L01 | 372.54 | 1 | 5 | 1 | 88.32 | Yes | 0 | 0.55 |
L02 | 385.56 | 2 | 5 | 0 | 85.16 | Yes | 0 | 0.55 |
L03 | 400.55 | 2 | 6 | 1 | 105.39 | Yes | 0 | 0.56 |
L04 | 373.57 | 1 | 5 | 1 | 94.11 | Yes | 0 | 0.55 |
L05 | 373.59 | 1 | 4 | 0 | 68.09 | Yes | 0 | 0.55 |
L06 | 389.62 | 2 | 5 | 1 | 94.11 | Yes | 0 | 0.55 |
L07 | N/A | N/A | N/A | N/A | N/A | N/A | N/A | N/A |
L08 | 420.65 | 3 | 6 | 1 | 91.56 | Yes | 0 | 0.55 |
L09 | 420.63 | 4 | 6 | 1 | 105.39 | Yes | 0 | 0.55 |
L10 | 404.58 | 3 | 6 | 1 | 105.39 | Yes | 0 | 0.55 |
L11 | 405.64 | 3 | 5 | 0 | 77.32 | Yes | 0 | 0.55 |
L12 | 417.65 | 3 | 5 | 0 | 85.16 | Yes | 0 | 0.55 |
Ligand | Absorption Human Intestinal | Caco-2 Permeability | Blood-Brain Barrier (BBB) | P- I Glycoprotein Inhibitor | Cation Transporter Renal Organic | P- II Glycoprotein Substrate | Sub-cellular Localization | Substrate CYP450 2C9 | Inhibitor CYP450 1A2 | |
---|---|---|---|---|---|---|---|---|---|---|
L01 | 0.7239 | 0.611 | 0.537 | 0.6175 | 0.562 | 0.8164 | 0.7225 | 0.8825 | 0.7848 | |
L02 | 0.9075 | 0.5354 | 0.7668 | 0.6114 | 0.5285 | 0.7211 | 0.7290 | 0.8840 | 0.6375 | |
L03 | 0.7019 | 0.5973 | 0.5344 | 0.9359 | 0.5000 | 0.7728 | 0.7673 | 0.8590 | 0.7245 | |
L04 | 0.9741 | 0.6125 | 0.5373 | 0.9118 | 0.6473 | 0.7913 | 0.4522 | 0.8890 | 0.6712 | |
L05 | 0.9790 | 0.5218 | 0.8720 | 0.7456 | 0.5000 | 0.7741 | 0.6092 | 0.8971 | 0.5491 | |
L06 | 0.9870 | 0.5983 | 0.8365 | 0.9643 | 0.5324 | 0.7949 | 0.4458 | 0.8986 | 0.5956 | |
L07 | 0.9878 | 0.5972 | 0.8475 | 0.6379 | 0.5969 | 0.8630 | 0.4340 | 0.9349 | 0.6489 | |
L08 | 0.8136 | 0.6144 | 0.5551 | 0.6313 | 0.6908 | 0.8643 | 0.4104 | 0.8941 | 0.7354 | |
L09 | 0.5487 | 0.6228 | 0.5949 | 0.6836 | 0.6176 | 0.8097 | 0.5974 | 0.8904 | 0.7455 | |
L10 | 0.7131 | 0.6216 | 0.6669 | 0.6864 | 0.5745 | 0.8252 | 0.5740 | 0.8581 | 0.7426 | |
L11 | 0.9102 | 0.5259 | 0.7580 | 0.8718 | 0.5254 | 0.8627 | 0.5518 | 0.9064 | 0.7167 | |
L12 | 0.9378 | 0.5309 | 0.7621 | 0.7862 | 0.5063 | 0.7819 | 0.6319 | 0.9109 | 0.7150 |
3.10. In Silico Analysis
3.10.1. Pharmacokinetics and Drug-likeness Study
The pharmacokinetics and drug-likeness study of the twelve ligands revealed important molecular characteristics relevant to their potential as drug candidates. Molecular weight ranged from 372.54 to 420.65 g/mol, within the typical range for small molecule drugs. The number of rotatable bonds ranged from 1 to 4, indicating moderate structural flexibility. Hydrogen bond acceptor and donor counts varied from 4 to 6 and 0 to 1, respectively, influencing the ligands' potential for interacting with target proteins. The topological polar surface area (TPSA) ranged from 68.09 to 105.39 Å2, influencing ligand solubility and permeability. Notably, Lipinski’s rule compliance was observed for all ligands, suggesting favorable drug-like properties. Furthermore, the bioavailability score indicated high bioavailability potential for all ligands, reinforcing their suitability as drug candidates, as mentioned in Table 8. Moreover, all ligands demonstrated a bioavailability score of 0.55, suggesting moderate bioavailability, and none violated Lipinski’s rule, further indicating their pharmacokinetic suitability for drug development. However, ligand L07 lacked complete data, limiting a full assessment. Overall, these findings suggest that the ligands exhibited promising physicochemical properties, making them suitable for therapeutic applications with effective oral bioavailability and pharmacokinetics.
3.10.2. Prediction of Toxicity Study
Table 9 presents a range of parameters related to drug toxicity, encompassing ligand absorption, human intestinal Caco-2 permeability, blood-brain barrier (BBB) permeability, P-glycoprotein inhibitor properties, cation transporter renal organic function, P-glycoprotein substrate specificity, sub-cellular localization, substrate activity towards CYP450 2C9, and inhibitor effects on CYP450 1A2. Ligand absorption data sheds light on the drug's assimilation within the body, with L04, L05, L06, L07, and L12 demonstrating elevated absorption rates. Human intestinal Caco-2 permeability values exhibit variance among the drugs, notably with L04, L08, and L09 displaying relatively higher permeability. Blood-brain barrier (BBB) permeability is particularly critical for drugs targeting the central nervous system, where L05, L06, and L07 exhibit heightened permeability. P-glycoprotein inhibitors play a crucial role in combating drug resistance, with L04 and L11 showcasing significant inhibitory potential. Cation transporter renal organic values are pivotal for drug excretion mechanisms, with L08, L09, and L10 demonstrating elevated values. Substrate CYP450 2C9 data holds importance in understanding drug metabolism pathways, with L08, L09, L10, and L12 emerging as noteworthy substrates. Inhibitor CYP450 1A2 values are crucial indicators of potential drug interactions, with L09, L10, and L12 displaying inhibitory effects.
In summary, L04, L05, L06, L07, and L12 showcase promising attributes across multiple toxicity parameters, rendering them potentially intriguing candidates for further exploration or drug development endeavors. However, a holistic examination considering all parameters collectively is indispensable to accurately assess their overall toxicity profiles.
3.11. Molecular Dynamics
3.11.1. Root Mean Square Deviation (RMSD)
To delve into the structural dynamics and validate the docking precision of the leading ligand-protein complexes involving PDB: 5GWK-L04 and PDB: 4OQ3-L1, we carried out 100 ns molecular dynamics. Through meticulous analysis of the C-α atom's RMSD, we assessed the resilience of these intricate complexes. The temporal evolution, spanning from 0 to 34 ns, was meticulously scrutinized along the x-axis, aptly labeled “Time (nanosecond),” while the y-axis, denoted as “Protein RMSD (Å),” indicating the root mean square displacement of the protein in angstroms (Å). It was found the RMSD values consistently lingered below the 2-3 Å threshold, indicative of an enduringly stable protein structure throughout the simulation period. This depiction underscores the robustness and reliability of the ligand-protein complexes under scrutiny, suggesting promising prospects for their pharmaceutical applications. As shown in Fig. 12(a), the RMSD starts at 0 and increases slightly over time, with a final value around 2.5 angstroms (Å) at 20 nanoseconds. This suggests that the protein structure undergoes small changes during the simulation. As shown in Fig. 12(b), the RMSD starts at around 2.2 angstroms (Å) and fluctuates slightly, staying around 2.2-2.7 Å throughout the 100ns simulation. This suggests that the protein-ligand complex undergoes small changes during the simulation but maintains a relatively stable structure overall.
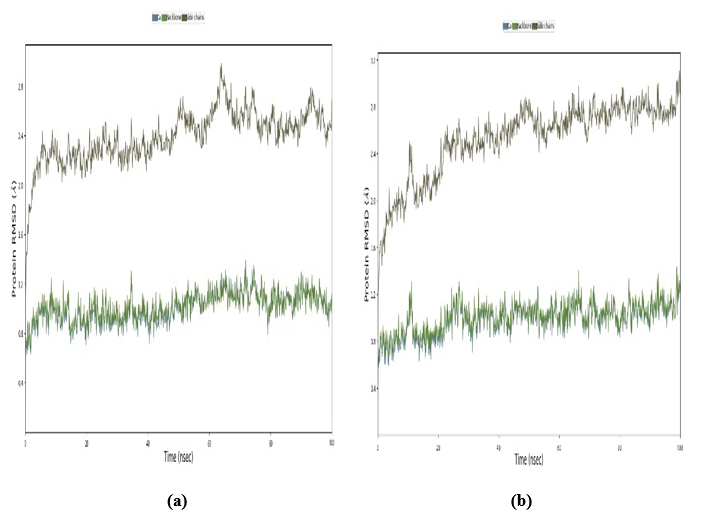
Root mean square deviation (RMSD): (a) PDB: 5GWK-L04 and (b) PDB: 4OQ3-L01.
3.11.2. Root Mean Square Fluctuation with Respect to Residues
Root Mean Square Fluctuation (RMSF) is a widely utilized metric in the analysis of MD simulations or experimental data pertaining to proteins or other biomolecules [52]. It offers valuable insights into the flexibility and mobility of individual amino acid residues within a protein structure. Mathematically, RMSF is computed as the square root of the average of the squared displacements (or fluctuations) of each residue from its mean position throughout a simulation or experiment. For a protein with N residues, the RMSF of residue i (RMSFi) can be expressed as the square root of the average of the squared deviations of each residue from its mean position [52] (Eq. 9).
![]() |
(9) |
Where n is the number of frames or snapshots in the simulation or experimental data, xij is the position of residue, i is in the frame j, and x̄i is the average position of residue i over all frames [52]. In addition, the high RMSF values for certain residues indicate that those residues are more flexible or dynamic. The low RMSF value suggests that those residues are more rigid or less dynamic. RMSF values can be correlated with structural features, functional regions, or interactions within the protein. For example, loops and flexible regions tend to have higher RMSF values compared to core secondary structure elements, such as α helices and beta strands. As shown in Fig. (13), the RMSF values are significantly lower, indicating that they do not exceed approximately 2.5 Å throughout the 100 ns simulation. An RMSD value of around 2.5 Å indicates that the docking predictions are fairly accurate, with only minor discrepancies between the predicted and actual ligand-protein interactions. This degree of variation is typically considered acceptable in virtual screening and early-stage drug design, where the primary goal is to identify potential ligand candidates for further refinement and optimization in subsequent studies. However, it is a valid procedure.
3.11.3. Beta Factor
In MD simulations, the term “beta factor” usually refers to the atomic or residue B-factor, also known as the atomic temperature factor. The B-factor is a measure of the mobility or flexibility of atoms or residues within a bimolecular system. It is particularly useful in understanding the dynamic behavior of proteins and other macromolecules. In MD simulations, B-factors can be calculated directly from the positional fluctuations of atoms or residues. The B-factor for an atom or residue is typically computed as the average over time of the squared displacement of that atom or residue from its mean position. High B-factors indicate greater mobility or flexibility of atoms or residues. Low B-factors suggest rigidity or restricted motion. B-factors can provide insights into regions of proteins that are structurally flexible or undergo conformational changes. A lower B factor can be seen in Fig. (14), which indicates the high stability.
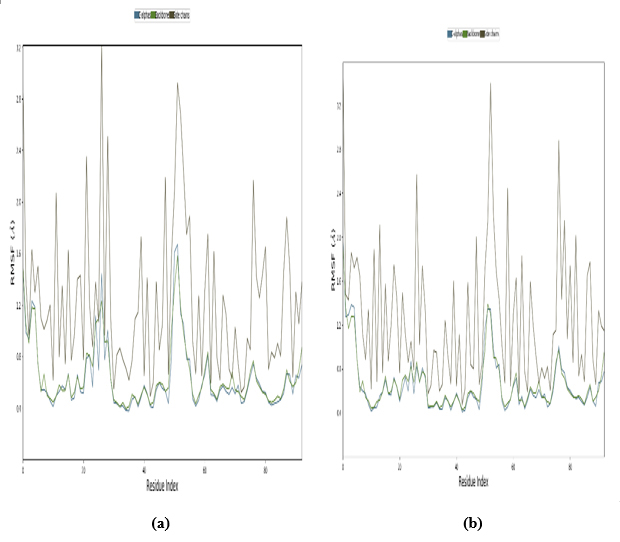
Root mean square fluctuation with respect to residues (a) PDB: 5GWK-L04 and (b) PDB: 4OQ3-L01.
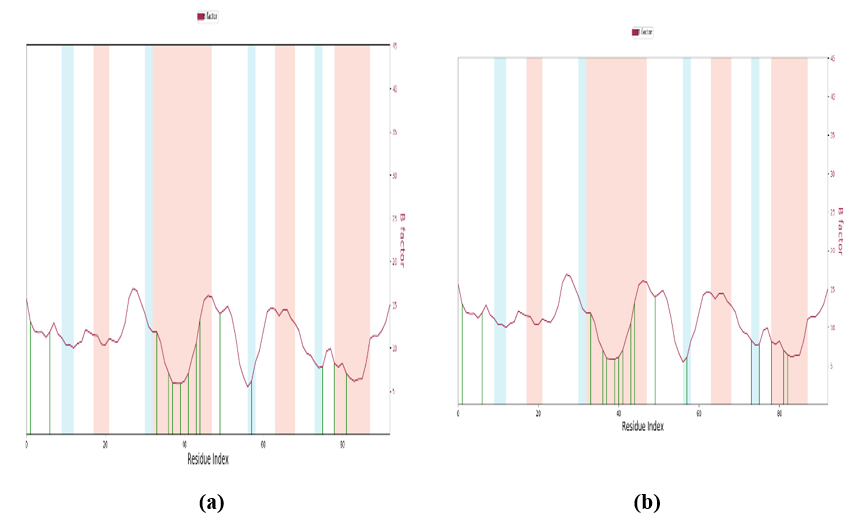
Beta factor: (a) PDB: 5GWK-L04 and (b) PDB: 4OQ3-L01.
The Beta factor, or B-factor, serves as a metric to gauge the flexibility and mobility of residues within protein structures during MD simulations. It measures the extent to which a specific residue oscillates or moves around its central position. Elevated B-factor values signify heightened flexibility, while lower values indicate rigidity. The binding of heavy metals, such as zinc, iron, or copper, to specific residues within proteins can induce significant alterations in their behavior, such as stabilization and destabilization. Certain heavy metals function as structural stabilizers by establishing coordination bonds with amino acid side chains. This interaction tends to decrease the flexibility of adjacent residues, thereby influencing the overall dynamics of the protein. Conversely, heavy metals may disrupt native interactions within the protein, leading to conformational changes. These modifications can propagate throughout the protein structure, affecting its functionality and stability. In essence, heavy metals exhibit a dual role in modulating protein dynamics, either enhancing or perturbing them depending on their binding sites and coordination. A comprehensive understanding of these effects is paramount for predicting protein behavior across diverse biological contexts. Additionally, both graph illustrates that the beta factor experiences minimal fluctuations, typically ranging between -0.2 and 0.2 for the majority of residues. This indicates that individual amino acids within this protein sequence have relatively little influence on the protein's stability in isolation.
3.11.4. Protein-ligand Interaction by Bond
Fig. (15) depicts two distinct graphical representations, each offering insights into different aspects of the data being analyzed. In the upper graph, a bar chart structure in Fig. (15 a,b) is employed, showcasing diverse categories or groups distinguished by contrasting colors, as outlined in the legend. Along the horizontal axis, various categorical labels or time points are delineated, while the vertical axis likely denotes a numerical measure, such as counts or quantitative values. The varying heights of the bars indicate fluctuations or disparities within the dataset across the specified categories.
In contrast, the lower graph presents a more intricate visualization comprising three distinct segments. At the top, a waveform pattern is discernible, suggesting a time-dependent measurement or trend. The middle section features a raster plot characterized by color-coded markings commonly utilized to illustrate spiking activity or categorical occurrences over a temporal continuum. Lastly, the bottom section incorporates a series of short vertical lines or markers, potentially indicative of specific events or temporal markers within the dataset.
Overall, these graphical representations offer a comprehensive depiction of the data, allowing for nuanced interpretation and analysis of the underlying trends and patterns present within the dataset.
3.11.5. Radius of Gyration (Rg) of WT, Mutations, and Solvent-Accessible Surface Area (SASA)
The radius of gyration for a particular system or object is equal to the square root of the moment of inertia divided by the total mass.
From the radius of gyration data, it was found that stability and response to external forces under different loading conditions of the structure are valid and stable. In MD simulations, the SASA is an essential metric that offers important details on how atoms in a bimolecular system are exposed to the surrounding solvent. SASA is frequently used to investigate how ligands and proteins interact. Variations in solvent accessibility can reveal areas that are revealed during unbinding or concealed during ligand binding. Finding binding locations and comprehending the energetics of protein-ligand interactions benefit from this. Data from MD simulations, along with the SASA analysis, helps to show that the working procedure of docking is valid by experimental data by providing insights into the dynamic behavior of molecules, revealing solvent exposure and flexibility that may not be evident in static structures, as shown in Fig. (16 a,b).
Fig. (16 a,b) displays two sets of charts. Protein-ligand complexes (a) PDB: 5GWK-L04 and (b) PDB: 4Q03-L01, each featuring three vertically arranged line graphs, likely represent time-series data. These charts correspond to distinct protein-ligand complexes identified by PDB codes 5GWK-L04 and 4Q03-L01, potentially referencing the Protein Data Bank. The top graphs indicate fluctuations around mean values, possibly reflecting biological or physicochemical parameters within the complexes. The middle graphs demonstrate erratic fluctuations, suggesting intermittent events or interactions, while the bottom graphs exhibit periodic variations, hinting at recurring structural changes or events. Color-coded bars at the bottom may denote experimental conditions, including indications like “No intermolecular HBs Detected,” implying the absence of intermolecular hydrogen bonds. While the observed patterns provide insights into the dynamics and stability profiles of the complexes, a detailed analysis would require higher-resolution images or supplementary data to elucidate the parameters measured and their significance.
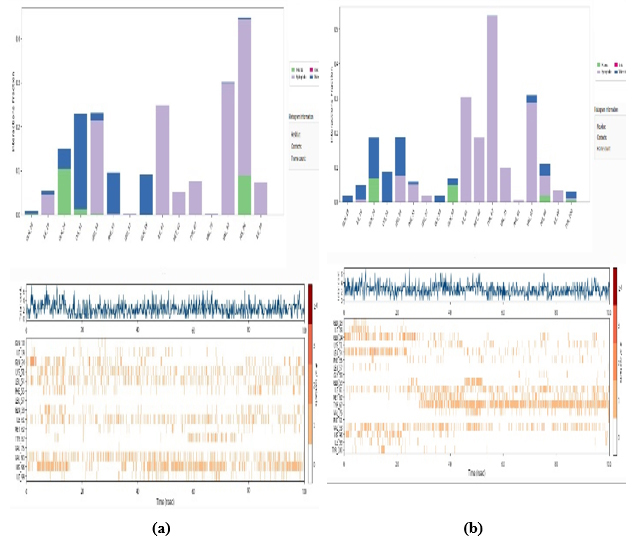
Protein-ligand interaction bond for (a) PDB: 5GWK-L04 and (b) PDB: 4OQ3-L01.
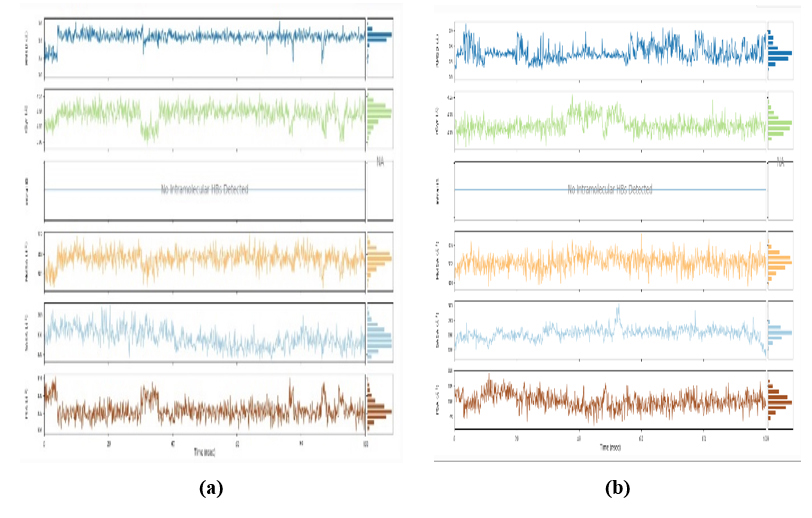
The radius of gyration (Rg) of WT, mutations, and solvent-accessible surface area (SASA) for (a) PDB: 5GWK-L04 and (b) PDB: 4OQ3-L01.
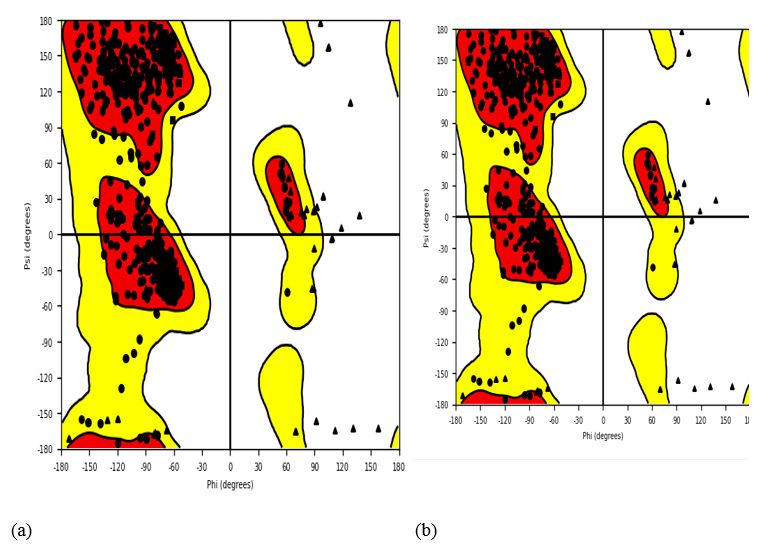
Ramachandran plot for docked protein complex. (a) PDB: 5GWK-L04 and (b) PDB:4OQ3-L01.
3.11.6. Ramachandran Plot for Docked Protein Complex
Embedded within the image, the Ramachandran plot emerges as a cornerstone in structural biology, presenting a graphical depiction of torsion angles, notably dihedral angles, within the protein's architecture. This grid, delineated by Phi (Φ) and Psi (Ψ) degrees along the x and y axes, respectively, spans the -180 to 180-degree range, as showcased in Fig. (17). Each dot adorning the plot corresponds to an amino acid residue within the protein, facilitating a thorough examination of its structural nuances. Marked areas discern α-helices and β-sheets, providing insights into the protein's folding patterns and conformation based on these torsional angles.
A close analysis of the plot reveals four distinct clusters of black dots, denoting data points for dihedral angles within the protein structure. Yellow zones highlight preferred combinations of Ψ and Φ angles typical for amino acid residues, with red sectors indicating highly favored combinations within these zones. Functioning as a compass for torsion angles, the Ramachandran plot is instrumental in unraveling the distribution of these angles and appraising the protein's three-dimensional arrangement. While theoretical forecasts offer average Φ and Ψ values for α-helices and β-sheets, experimental structures often veer off course due to diverse influences. These deviations shape the clustering patterns of dots on the plot, serving as indicators of the protein structure's fidelity. In essence, the Ramachandran plot furnishes invaluable insights into the protein's conformation, delineating permissible and prohibited regions of torsion angle values, thus playing an indispensable role in scrutinizing protein structural integrity.
CONCLUSION
First of all, DFT calculations demonstrated that ligands with narrower HOMO-LUMO gaps, like L03 and L04, were more reactive, while those with wider gaps, like L05, were more stable and electron-accepting. In addition, the analysis of electrostatic potential maps revealed that varying charge distributions in molecules influenced reactivity and selectivity, highlighting the complexity of predicting binding mechanisms and designing molecules with specific properties. Next, in this extensive investigation, the comparative analysis of binding energies obtained from PyRx and Glide molecular docking simulations of twelve ligands with human topoisomerase IIα (5GWK) and p53 (4OQ3) provided insights into the efficacy and ability of drug potential by computational tools for TNBC as well as in silico study. Both methods demonstrated similar trends in ligand affinities for both protein targets, with notable variations in absolute values. Ligands consistently exhibited higher binding affinities with 5GWK compared to p53 across both docking platforms. However, there were discrepancies in binding energies between PyRx and Glide docking for some ligands, suggesting differences in their scoring functions and algorithms. Ligands L01, L02, and L09 showed relatively higher binding energies with 5GWK in PyRx compared to Glide docking, while ligands L07 and L08 displayed the opposite trend. Both Glide docking and AutoDock demonstrated consistent binding trends for ligands against 5GWK and 4OQ3 proteins, with L05 as a strong binder for 5GWK. Hydrogen and hydrophobic interactions are crucial, emphasizing the value of these methods in designing dual-target anticancer agents. In silico studies identified promising toxicity profiles (drugs L04, L05, L06, L07, and L12), making them strong candidates for further exploration, but a comprehensive evaluation of all parameters is essential for accurate toxicity assessment. These variations underscore the importance of considering multiple docking methods to obtain comprehensive insights into ligand-protein interactions. The final findings highlighted that ligands with narrower HOMO-LUMO gaps showed higher reactivity, while those with wider gaps, like L05, offered greater stability, with computational tools providing valuable insights into binding affinities, toxicity, and dual-target anticancer drug design, offering valuable insights for the rational design of more effective anticancer agents targeting TNBC.
AUTHORS’ CONTRIBUTIONS
Z., B. and M: Study Concept or Design; M., F.: Data Analysis or Interpretation; H.: Writing the Paper; A., N., S.: Study Concept or Design; M.: Writing - Original Draft Preparation.
LIST OF ABBREVIATIONS
CPT | = Camptothecin |
TNBC | = Triple-negative breast cancer |
ER | = Estrogen receptor |
PR | = Progesterone receptor |
HER2 | = Human epidermal growth factor receptor 2 |
CPT | = Camptothecin |
TNBC | = Triple-negative breast cancer |
PDB | = Protein data bank |
HBD | = Hydrogen bond donors |
HBA | = Hydrogen bond acceptors |
AVAILABILITY OF DATA AND MATERIALS
The data and supportive information are available within the article.
ACKNOWLEDGEMENTS
The authors would like to express their thanks to the IUBAT Innovation and Entrepreneurship center, IUBAT—International University of Business Agriculture and Technology, 4-Embankment Drive Road, Sector 10 Uttara Model Town, Dhaka 1230, Bangladesh, for the technical support provided to carry out this research.